<Understanding AI Bias: Merging Foundational Principles with Systems Thinking>
Written on
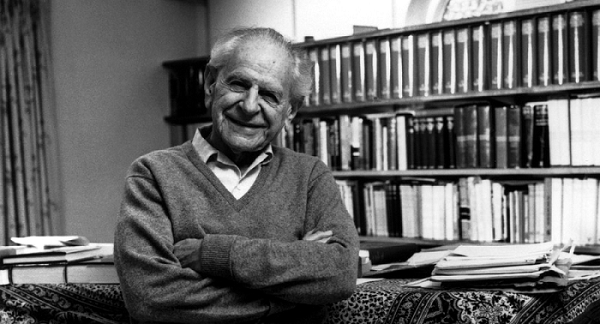
Over time, I have contemplated the nature of AI deeply.
We possess the ability to replicate or outsource elements of our intelligence in a non-biological way. This capacity captivates people from various backgrounds and gives AI the potential to influence us all.
A quote from philosopher Karl Popper has lingered in my mind for years:
“I believe there is only one path to science — or philosophy: to confront a challenge, appreciate its beauty, become devoted to it, and engage with it until a solution is found. Even then, one might uncover an entire family of intriguing problems that could occupy one’s efforts for a lifetime.”
AI is that captivating challenge for me. As I delve deeper, its complexities continue to enchant me. Consequently, I strive to refine the analytical tools I use to explore this technology and its potential effects on society.
Throughout my journey with AI, two philosophical frameworks have emerged as particularly significant: First Principles thinking and Systems Thinking.
Foundational Thinking: First Principles and AI
First Principles thinking is an ancient concept, notably advanced by philosophers such as Aristotle.
Aristotle and his rationalist contemporaries argued that to truly understand and solve a problem, one must deconstruct it into its fundamental components.
This philosophy has been embraced by various intellectuals over time, and modern entrepreneurs like Elon Musk have popularized it. In the realm of AI, First Principles thinking is crucial. We need clarity rather than hype.
In AI, First Principles compel us to simplify and ask essential questions. Let’s consider a few of these:
What constitutes intelligence?
At its essence, intelligence is the capacity to acquire, comprehend, and apply knowledge. It manifests as reasoning, problem-solving, abstract thinking, understanding complex concepts, and adaptability to new situations. For humans, intelligence encompasses not only raw computation but also perception, judgment, and the synthesis of diverse information.
How do humans learn, and how might machines emulate or enhance this process?
Humans learn through experience, observation, and contemplation. When faced with new information or situations, we adapt our existing knowledge to integrate this new data, a process Aristotle referred to as “empirical learning.”
Conversely, machines, particularly those employing AI, learn by analyzing vast datasets, recognizing patterns, and adjusting their algorithms based on feedback. While they may lack human intuition and contextual understanding, they excel at processing large quantities of data, thereby complementing human learning.
What factors limit decision-making, whether human or machine-driven?
For humans:
- Knowledge limitations: We cannot know everything.
- Cognitive biases: Innate or learned inclinations can distort our judgments.
- Emotional influences: Emotions can obscure or direct our decision-making.
- Resource constraints: Decisions often occur under limitations of time and resources.
For machines:
- Data quality: Their decisions are contingent on the quality of the data they receive.
- Algorithm constraints: They operate strictly within their programmed parameters.
- Absence of context: Machines lack the human ability to interpret subtleties or instinctively grasp situations.
- Hardware limitations: Machines can only process data at their design capacity.
What is the fundamental essence and goal of AI?
At its core, AI is a human-engineered tool, derived from our understanding of intelligence, intended to process information and make decisions based on algorithms. Its purposes include:
- Enhancing human capabilities by automating challenging or tedious tasks.
- Rapidly analyzing extensive datasets to provide insights beyond human capacity.
- Simulating human-like functions across various domains, from gaming to medical diagnosis.
It’s essential to note that while AI can imitate certain aspects of human intelligence, it does not possess consciousness or emotions.
Implementing First Principles to Tackle AI Bias
Let’s further examine a practical issue in AI: bias in hiring practices. Below are guiding questions and responses based on these principles.
What defines a fair and unbiased hiring process?
Start by articulating the ideal outcome, free from bias. Understand the key elements that determine a candidate’s suitability, emphasizing skills and competencies rather than irrelevant characteristics.
A fair hiring decision evaluates candidates based on relevant qualifications and potential contributions to the role, avoiding unrelated factors like gender or race.
What are the sources and types of bias in hiring?
Investigate the origins of biases, recognizing both explicit and implicit forms. Explore how societal and personal beliefs can shape decision-making and how these biases might inadvertently be integrated into algorithms.
Biases in hiring can stem from:
- Societal stereotypes: Cultural beliefs can skew perceptions of suitability for certain roles.
- Previous experiences: Favoritism towards candidates from specific backgrounds due to positive prior interactions.
- Implicit biases: Unconscious assumptions affecting judgment, such as evaluating accents.
- Data bias: AI trained on skewed datasets may perpetuate existing inequalities.
- Flawed algorithms: Algorithms may inadvertently prioritize certain features, leading to biased outcomes.
How do AI-driven hiring systems process data to make decisions?
Analyze the technical aspects, including the data sources and how decisions are derived from them. Understanding this will help identify points where bias may be introduced.
AI hiring systems typically:
- Acquire Data from resumes, profiles, assessments, and social media.
- Process Data with algorithms to identify patterns and rank candidates. Natural Language Processing may analyze resumes, while scoring algorithms assess qualifications.
- Utilize Data to present a shortlist to recruiters, predict candidate success, or automate initial screenings.
What strategies can mitigate bias in AI hiring tools?
Based on the understanding of fair hiring, sources of bias, and AI operations, consider solutions like diverse datasets, transparent algorithms, continuous feedback, and independent audits.
Potential strategies include:
- Diverse training data: Ensuring representation from various backgrounds in training datasets.
- Algorithm transparency: Making algorithms interpretable to identify biases.
- Feedback mechanisms: Regularly comparing AI predictions with real outcomes to refine algorithms.
- Bias audits: Periodic evaluations by third parties to detect and address biases.
- Human oversight: Maintaining human involvement to provide additional scrutiny to AI recommendations.
- Education and training: Equipping developers and users with awareness of biases and mitigation strategies.
Integrating First Principles with Systems Thinking
While First Principles clarify the individual components, Systems Thinking helps us grasp their interconnections.
AI is not an isolated entity; it is part of a complex web involving human interactions, societal standards, and economic factors.
I became acquainted with Systems Thinking through the influential work of philosopher Fritjof Capra.
Systems Thinking is a comprehensive analytical approach that emphasizes understanding how parts of a system interrelate and function over time within larger contexts. Instead of addressing isolated components, Systems Thinking seeks to comprehend entire systems by examining the interdependencies among their parts. This perspective acknowledges that the whole is greater than the sum of its parts, and changes in one part can yield unforeseen effects throughout the system.
Capra highlighted the interconnectedness of biological, cognitive, and social dimensions within a broader ecological system. Embracing a systems view means recognizing the potential ripple effects of AI solutions. A modification in one area can lead to unforeseen consequences in others, reminding us to innovate ethically and sustainably.
Addressing AI Bias through a Systems Perspective
Combining First Principles and Systems Thinking provides an effective framework for tackling AI bias. First Principles allow us to dissect AI bias into its fundamental truths, challenging assumptions and establishing a foundational understanding. This approach ensures we address the root causes of bias rather than merely treating its symptoms.
Conversely, Systems Thinking enables us to visualize the larger landscape in which AI operates, mapping the complex web of interactions that can amplify or alleviate bias. By recognizing how various system components, such as societal norms and data sources, interact, we can identify intervention points.
The synergy of these two approaches offers a comprehensive understanding of the issue and a holistic view of its context. This dual perspective empowers us to develop solutions that are insightful and impactful, ensuring AI functions fairly within the broader ecosystem.
AI, as a technological construct, is intricately woven into the socio-technical fabric of our society. Bias in AI is not merely a coding error; it reflects broader societal prejudices, economic structures, historical contexts, and human psychology. From a systems perspective, several factors come into play:
- Training Data as a Reflection of Society: If certain demographics are underrepresented in data, AI systems may perpetuate these disparities. This is not solely a technological issue; it mirrors educational systems and corporate cultures.
- Economic Influences: If a biased AI system proves profitable, there may be less motivation to rectify its flaws. Market dynamics, regulatory frameworks, and societal awareness are crucial components of this landscape.
- Feedback Loops that Intensify Bias: Biased AI decisions can generate skewed data, creating a self-reinforcing cycle.
Step-by-Step Approach to Mitigating AI Bias with First Principles and Systems Thinking
Identify the Core Problem with First Principles: - Action: Dispel existing assumptions about AI bias. - Result: We may discover that the fundamental issue relates not just to algorithms but also to the training data.
Expand with Systems Thinking: - Action: Trace the origins of this data. - Result: We may find that training data often derives from historical records steeped in societal biases.
Isolate Key Variables with First Principles: - Action: Deconstruct the components of AI training data. - Result: Identify specific data subsets likely to perpetuate bias, such as hiring data from gender-imbalanced industries.
Analyze Feedback Loops with Systems Thinking: - Action: Investigate how biased AI decisions might influence future data. - Result: Recognize that biased AI in hiring can create skewed workplaces, further distorting future hiring data.
Craft Solutions Using First Principles: - Action: Focus on modifying the core problematic components. - Result: Introduce more diverse training data to ensure representation of the broader population.
Integrate and Test Solutions within the System: - Action: Implement the revised AI within the larger ecosystem, monitoring its interactions. - Result: Observe how the AI’s decisions impact the workplace over time, making adjustments to ensure fairness.
Iterate and Refine: - Action: Continually revisit the AI’s foundational principles and its role in the broader context. - Result: Develop a dynamic AI tool that aligns with societal values and remains resilient to biases.
By balancing the detailed focus of First Principles with the expansive lens of Systems Thinking, we establish a comprehensive strategy for effectively addressing AI bias.
Future articles will explore additional pressing issues in AI through these analytical frameworks.