Insights from Richard Feynman on Artificial General Intelligence
Written on
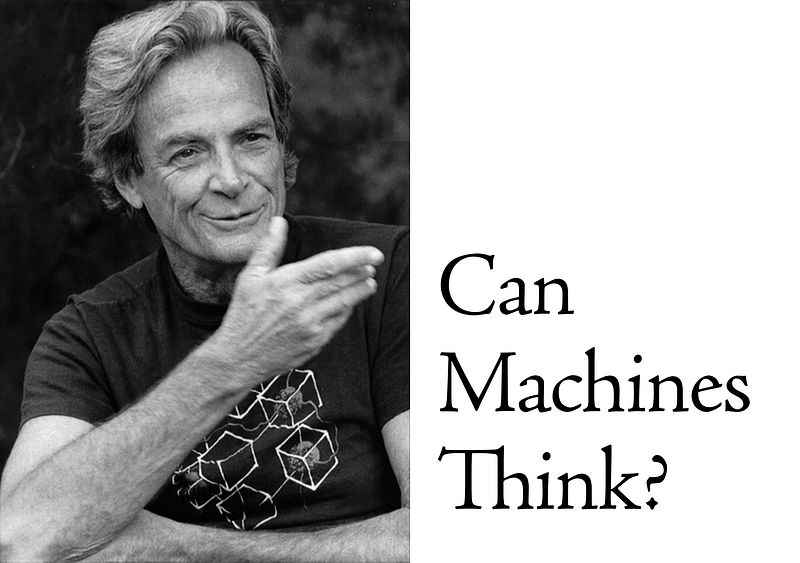
Artificial General Intelligence was a key topic in a lecture by Nobel Prize winner Richard Feynman (1918–1988) on September 26, 1985.
Audience Inquiry
An audience member posed the question: "Do you believe there will ever be a machine that thinks like humans and surpasses human intelligence?" Below is a detailed account of Feynman's response. Given the rise of machine learning through artificial neural networks, it’s intriguing to consider Feynman’s perspective from 35 years ago.
Estimated reading time: 8 minutes. Enjoy!
Feynman's Response
Feynman began by addressing the notion of whether machines can think like humans:
"First of all, do they think like human beings? I would say no, and I’ll explain in a moment why I say no."
He continued by discussing the criteria for defining intelligence:
"For the question of whether they could be more intelligent than humans to even arise, we must first define what intelligence means. If you were to ask if they are better chess players than humans, they possibly can be."
In 1985, human chess grandmasters still dominated machines. It wasn't until the famous six-game matches between world champion GM Garry Kasparov and IBM’s Deep Blue in 1996 and 1997 that a computer triumphed over a world-class player. Even then, the match concluded with a narrow victory, and Kasparov contested the outcome, alleging interference by the IBM team.
The AI Effect
Feynman then tackled the phenomenon known as the "AI effect," highlighting the tendency of observers to downplay a machine's abilities once it successfully accomplishes a task:
"They’re better chess players than most humans right now! We often expect a machine to outperform everyone, not just us. If we find a machine that excels at chess, it doesn’t impress us as much. We ponder, 'What happens when it faces the masters?' We presume that we humans equate to the masters in every domain. Thus, the machine must excel at everything the best can do."
Building Artificial Intelligence
Feynman further explored the concept of mental models, contrasting natural locomotion—like a mammal's running gait—with mechanically engineered movement:
"Regarding whether we can make machines think like humans, my view is shaped by the idea that we strive to create these systems to operate as efficiently as possible with the materials we have. Materials differ from nerves, and so forth. If we wish to design something that moves swiftly, we might observe a cheetah. However, it’s simpler to construct a machine with wheels or something that flies. Airplanes don’t mimic birds exactly; they don’t flap wings but utilize jet propulsion and other technologies. Clearly, later machines won't think like humans in that sense."
He elaborated on intelligence, noting:
"Machines won't perform arithmetic in the same manner as humans, but they will do it better."
Superhuman Narrow AI
Feynman illustrated the superiority of mechanical systems over human capabilities, particularly in basic mathematics:
"Consider elementary arithmetic. Machines execute it faster and in a fundamentally different way, but the end results are equivalent. We won’t change how they perform arithmetic to resemble human methods; that would be regressive. Human arithmetic is slow and prone to errors, while machines are swift."
He elaborated further:
"If I ask a human to provide every other number from a sequence in reverse order, it’s a challenging task, even with a list of twenty or thirty numbers. Yet, a computer can handle fifty thousand numbers without breaking a sweat."
Recognizing Patterns
Feynman moved closer to discussing the challenges of pattern recognition, a problem later addressed by supervised machine learning:
"Humans continually seek to find tasks they can execute better than computers. For instance, recognizing a familiar person by their distinctive walk or hairstyle is something we do naturally."
He expressed the difficulty in defining a procedure for recognition:
"You might say, 'I have a good method for recognizing a jacket: just take numerous pictures of Jack.' However, recognizing someone under various conditions is complex."
The Bias-Variance Tradeoff
Feynman then touched upon the challenges of variance in training data sets, which relates to the bias-variance tradeoff in machine learning:
"The issue arises when the actual conditions differ. Variations in lighting, distance, or angles complicate the recognition process. Even with powerful machines, creating a reliable procedure remains elusive."
He concluded:
"Recognizing patterns is challenging for machines, while humans can perform these tasks almost effortlessly."
The Current State of AI (1985)
In his final remarks, Feynman reflected on the complexities of designing machines for fingerprint matching:
"It seems simple to compare fingerprints, but numerous factors complicate matters. Different angles, pressures, and dirt can obscure the comparison, making it impractical for machines."
He acknowledged the rapid advancements in AI:
"While I’m unsure where they stand, progress is swift. Humans can navigate these complexities intuitively, unlike machines that struggle to recognize patterns quickly."
Video
You can view the complete video of Feynman's response at the link below:
This essay is part of a series exploring mathematics-related topics, featured in Cantor’s Paradise, a weekly Medium publication. Thank you for reading!